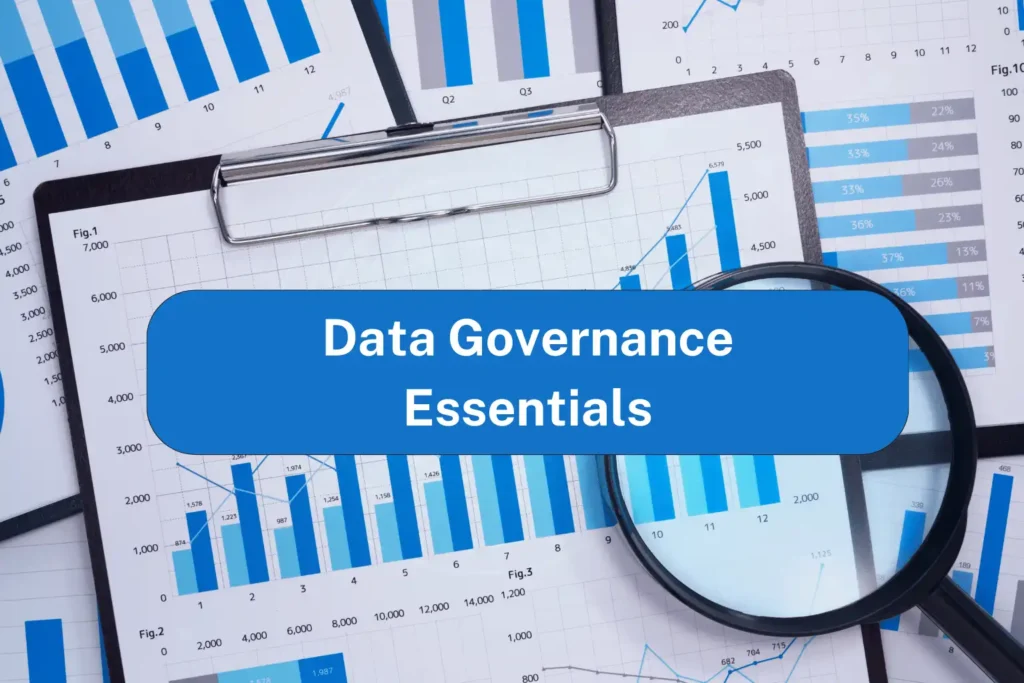
In today’s data-driven business environment, data governance has become a critical priority. As organizations leverage data science, machine learning, and AI for insights and decision-making, a robust data governance framework ensures that data remains accurate, accessible, and secure. This framework not only supports regulatory compliance but also fosters a culture of accountability and trust—key aspects of a data-driven organization.
For readers new to these concepts, you may find it helpful to review our AI fundamentals article, which explains how AI and data governance intersect to enable safe and ethical data use.
This article will explore data governance, its key components, and actionable steps for implementing an effective data governance framework that aligns with organizational goals.
What is Data Governance?
Data governance is a set of principles, policies, and practices that ensure data is managed consistently and responsibly throughout its lifecycle. It involves setting standards for data quality, security, and accessibility while assigning clear responsibilities for data assets.
In simpler terms, data governance creates a framework for reliable and secure data management, supporting compliance with data protection laws like GDPR and CCPA. For a more comprehensive look at these and other regulations, check out our Data Compliance article, which covers key regulations and why they’re crucial to businesses today.
Why Data Governance Matters
Data governance offers numerous benefits that contribute to a company’s ability to leverage data strategically and securely:
- Improved Data Quality: Data governance ensures data is accurate, complete, and consistent, making it useful for analytics and decision-making.
- Regulatory Compliance and Security: With growing data privacy regulations like GDPR and CCPA, organizations must govern data effectively to stay compliant and avoid penalties. Learn more about these regulations in our Data Compliance article.
- Data Accountability and Transparency: Clearly defined roles and policies enable organizations to track data ownership, promoting accountability and reducing risks.
- Cost Efficiency: A well-governed data ecosystem reduces redundant data storage and minimizes errors, saving time and resources.
Key Components of a Data Governance Framework
A robust data governance framework relies on several essential components that work together to create a cohesive approach to data management.
1. Data Governance Policies
Policies are the backbone of any data governance framework. They outline standards for data handling, access, sharing, and usage within the organization. Policies should align with business goals, regulatory requirements, and industry standards. Clear policies help guide employees at all levels in managing data responsibly and transparently.
2. Data Ownership and Stewardship
Defining roles, such as data owners and data stewards, establishes accountability for data across the organization. Data owners oversee specific data assets, while data stewards are responsible for ensuring data quality and adherence to governance policies. This clear delineation of responsibilities minimizes confusion and enhances accountability.
3. Data Quality Management
Data quality is a cornerstone of effective data governance. A data governance framework should include processes to monitor and maintain data accuracy, completeness, and consistency. Data quality management involves setting standards, running data profiling, and implementing validation checks to ensure data supports reliable insights.
4. Data Security and Privacy
With data breaches on the rise, data security and privacy are non-negotiable. Data governance frameworks must include security protocols such as encryption, access controls, and regular audits to protect sensitive information from unauthorized access. Ensuring compliance with privacy standards, such as those covered in our Data Compliance article, builds trust with customers and regulators alike.
5. Data Access Controls
Access controls are critical to managing who can view, modify, and share data. Role-based access controls (RBAC) are a common strategy, granting permissions based on an employee’s role and ensuring that sensitive data is only accessible to authorized personnel. Effective access controls protect against unauthorized data access while providing necessary data availability.
6. Data Lifecycle Management
Data governance must address the entire data lifecycle, from creation to disposal. Data lifecycle management involves policies on data retention, archival, and secure disposal to ensure that data is stored only as long as necessary. Effective lifecycle management reduces storage costs, maintains data relevance, and ensures compliance with retention laws.
Establishing Roles and Responsibilities
An effective data governance framework requires collaboration across departments. The following roles play a key part in data governance:
- Data Governance Council: This group oversees the governance framework, sets priorities, approves policies, and ensures alignment with organizational goals.
- Data Owners and Stewards: Data owners have control over specific datasets, while data stewards maintain data quality and compliance with standards.
- Data Custodians: Often part of IT, custodians handle the technical infrastructure, ensuring data is available and secure.
- Executive Sponsors: Executive backing is crucial for successful governance. When leaders champion data governance, it reinforces the organization’s commitment to data integrity.
Implementing Data Governance Processes
Implementing a data governance framework can be complex, but following these steps simplifies the process and helps maintain long-term compliance and accountability.
- Policy Creation and Documentation: Begin by defining policies for data use, privacy, and security. Documenting these policies provides a reference that all employees can understand and follow.
- Data Quality Standards and Monitoring: Establish metrics for data quality, such as accuracy, completeness, and timeliness. Implement data profiling and validation processes to ensure data consistently meets these standards.
- Data Access and Security Protocols: Set up robust access controls to protect data. Use encryption and access control solutions to secure sensitive information while allowing appropriate access to authorized users.
- Data Lifecycle Management: Develop processes for data retention, archival, and deletion. These processes maintain data relevancy, ensure compliance with retention laws, and optimize storage resources.
Tools and Technologies for Data Governance
Technology is essential for effective data governance. The following tools streamline governance efforts and help organizations manage data more efficiently:
- Data Catalogs: These tools support data discovery and metadata management, making it easier for users to find and understand data assets. For a deeper understanding of how data catalogs enhance AI and data use, see our AI fundamentals article.
- Data Lineage Tools: Tracking data lineage reveals how data flows through systems and applications, promoting transparency and enabling error tracking.
- Data Quality Tools: Automated tools for data profiling, cleansing, and validation ensure data accuracy and reliability.
- Access Management Solutions: Access control tools enforce security policies by managing user permissions and monitoring data access logs.
Measuring and Monitoring Data Governance Success
Monitoring and evaluating data governance efforts is essential for ensuring long-term success. Key performance indicators (KPIs) for data governance can include:
- Data Quality Metrics: Measure data accuracy, completeness, and consistency.
- Compliance Audits: Conduct regular audits to verify compliance with data governance policies and external regulations.
- Policy Adherence: Track employee adherence to data governance policies through audit logs and compliance monitoring tools.
By monitoring these metrics, organizations can identify areas for improvement and adapt governance practices as their data needs evolve. For further insights on compliance metrics and strategies, refer to our Data Compliance article.
Challenges in Building a Data Governance Framework
Data governance implementation can come with challenges. Some of the most common issues include:
- Cultural Resistance: Employees may be resistant to new policies or changes, making change management crucial to adoption.
- Resource Limitations: Smaller businesses may struggle to allocate the necessary resources—time, budget, and expertise—for effective data governance.
- Data Silos: Data stored in separate systems can create silos, making it difficult to enforce consistent governance.
- Evolving Regulations: With regulations continually changing, organizations must stay current with updates to ensure compliance. For more on regulatory trends, explore our Data Compliance article.
Best Practices for Data Accountability in Data Governance
To create a successful data governance framework, organizations should adhere to these best practices:
- Transparency in Data Policies: Make governance policies accessible and understandable for all stakeholders.
- Consistent Training and Awareness Programs: Conduct regular training sessions to keep employees informed about data governance practices.
- Continuous Improvement: Regularly review and update governance practices to adapt to regulatory changes and new business needs.
Conclusion
A well-structured data governance framework is essential for data accountability and reliability. By establishing robust policies, defining clear roles, and using the right tools, organizations can build a foundation for secure, compliant, and high-quality data management. Investing in data governance not only supports regulatory compliance but also fosters a culture of accountability, empowering businesses to use data responsibly and effectively.